Drug and Medical Technology Evaluation
Quick Links
Optimizing Satellite Constellations: A Case Study on ATS MDP Model Application
Probabilistic Risk Analysis of a Substation
Predictive Asset Management
Hospital Operations and Personnel Management
- Case Study 6
Drug and Medical Technology Evaluation
Pandemic Preparedness and Response
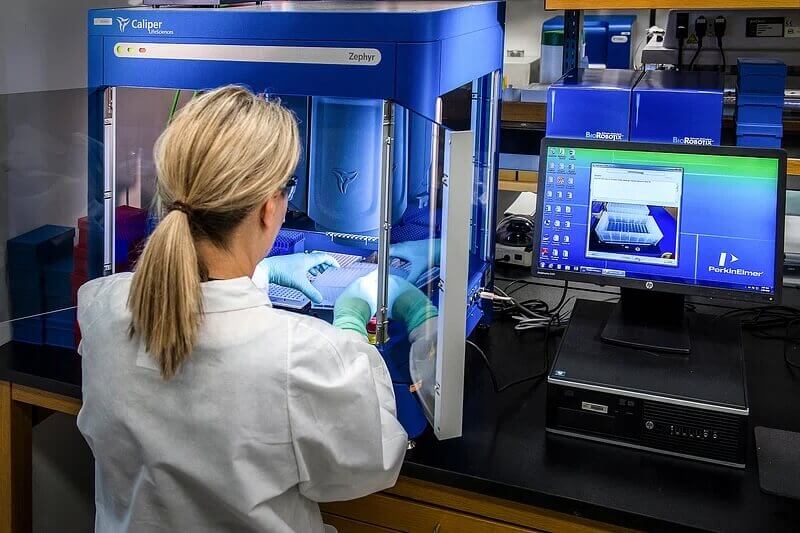
ATS specializes in developing data-driven mathematical and artificial intelligence models for health economic evaluations, such as cost-effectiveness analyses, for newly developed drugs or technology.
Demonstrating the cost-effectiveness and value of the drug or technology to payers, healthcare providers, and other stakeholders to secure reimbursement and ensure market access. Health economic evaluations play a crucial role in reimbursement decisions by demonstrating the economic viability and affordability of the intervention within healthcare systems.
ATS is an innovator in cost-effectiveness methodology providing tailored solutions based on machine learning and artificial intelligence ensuring that our analyses are second to none.
Facing recurrent outbreaks of plague, Madagascar sought guidance on allocating resources between preventive measures and conventional treatment. With limited funds and competing priorities, they required evidence-based recommendations to optimize public health outcomes while maximizing cost-effectiveness.
Challenges
ATS identified challenges included:

Resource Allocation Dilemma:
Balancing investments between preventive interventions, such as mass prophylaxis, and curative treatments, like antibiotics, presented a complex decision-making challenge.
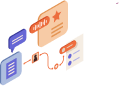
Uncertainty in Cost-Effectiveness:
Existing uncertainties regarding the cost-effectiveness of different intervention strategies required robust analytical frameworks to inform evidence-based decision-making.

Real-Time Decision Support:
The need for rapid, reliable analyses during outbreaks necessitated the development of a flexible and adaptive modeling approach to provide timely recommendations to decision-makers.
ATS leveraged its expertise in health economic evaluations and innovative methodologies to address these challenges in several ways:
Customized Disease Model: ATS developed a disease model tailored to the unique epidemiological and social dynamics of plague outbreaks in Madagascar. This model captured the transmission dynamics, healthcare utilization patterns, and economic costs associated with different intervention strategies.
Integration of Cost Data: Comprehensive cost data, including administration costs, drug costs, and inpatient hospital costs, were incorporated into the disease model to assess the economic implications of various intervention options accurately.
Decision Analysis Framework: Using a decision analysis and cost-effectiveness framework, ATS evaluated the trade-offs between costs and health benefits (measured in Quality-Adjusted Life Years) associated with preventive mass prophylaxis versus conventional treatment.
Machine Learning Integration: To enhance the scalability and utility of the model for real-time decision support, ATS developed a machine learning-based algorithm. This algorithm identifies the most cost-effective intervention strategy based on evolving disease dynamics and cost inputs, enabling on-demand recommendations during outbreaks.
Based on the cost-effectiveness analysis, ATS recommended investing solely in expanding access to conventional treatment, as it proved to be both cost-effective and affordable compared to mass prophylaxis.
To improve preparedness for future outbreaks, we developed a machine learning-based model which identifies the cost-effective intervention strategy as a function of disease dynamics and drug costs allowing decision makers to have access to on-demand recommendations in the midst of an outbreak.
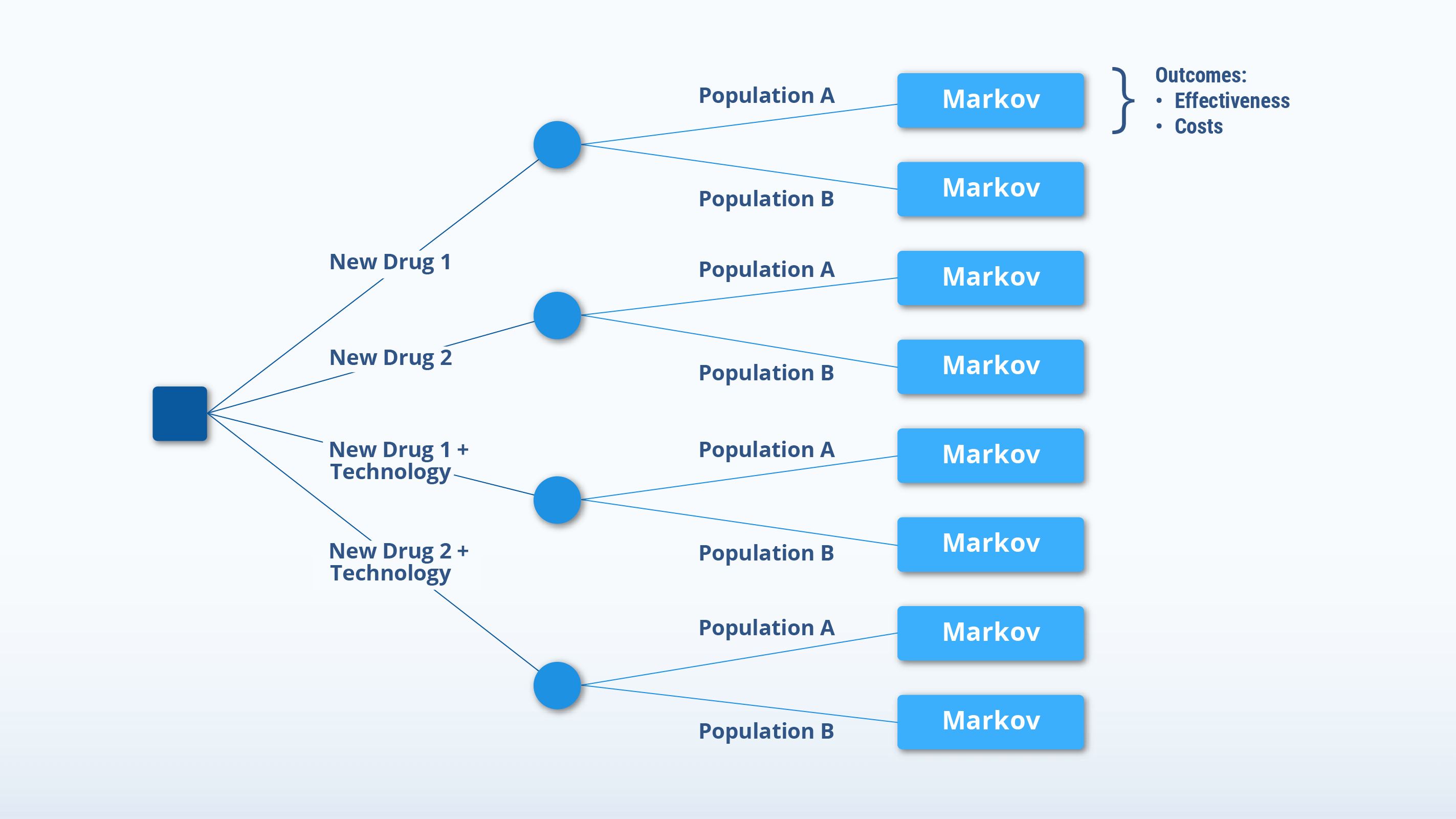
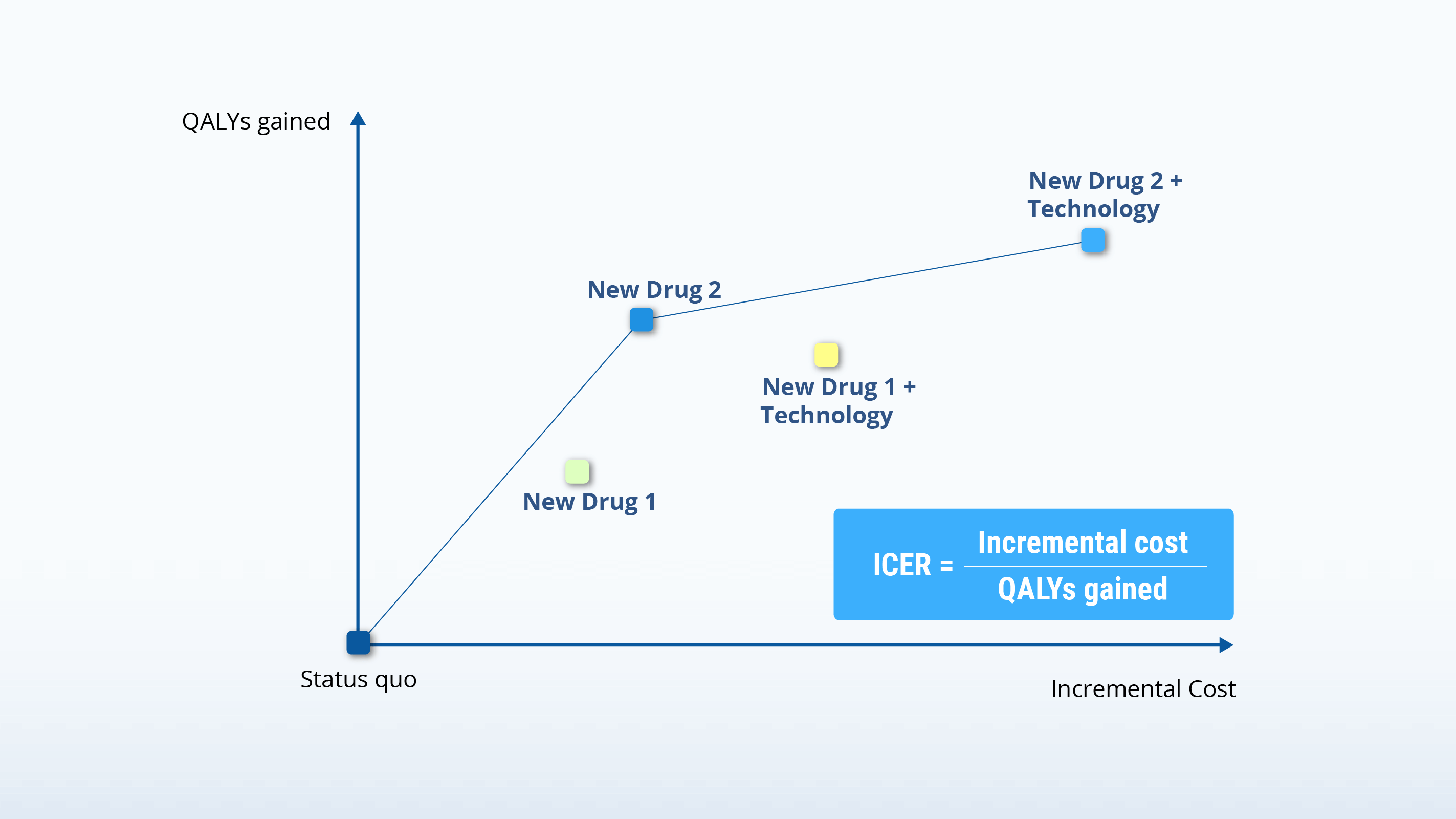